RavenPack uses 2iQ’s insider transaction data to create Alpha-Generating strategies across European Markets
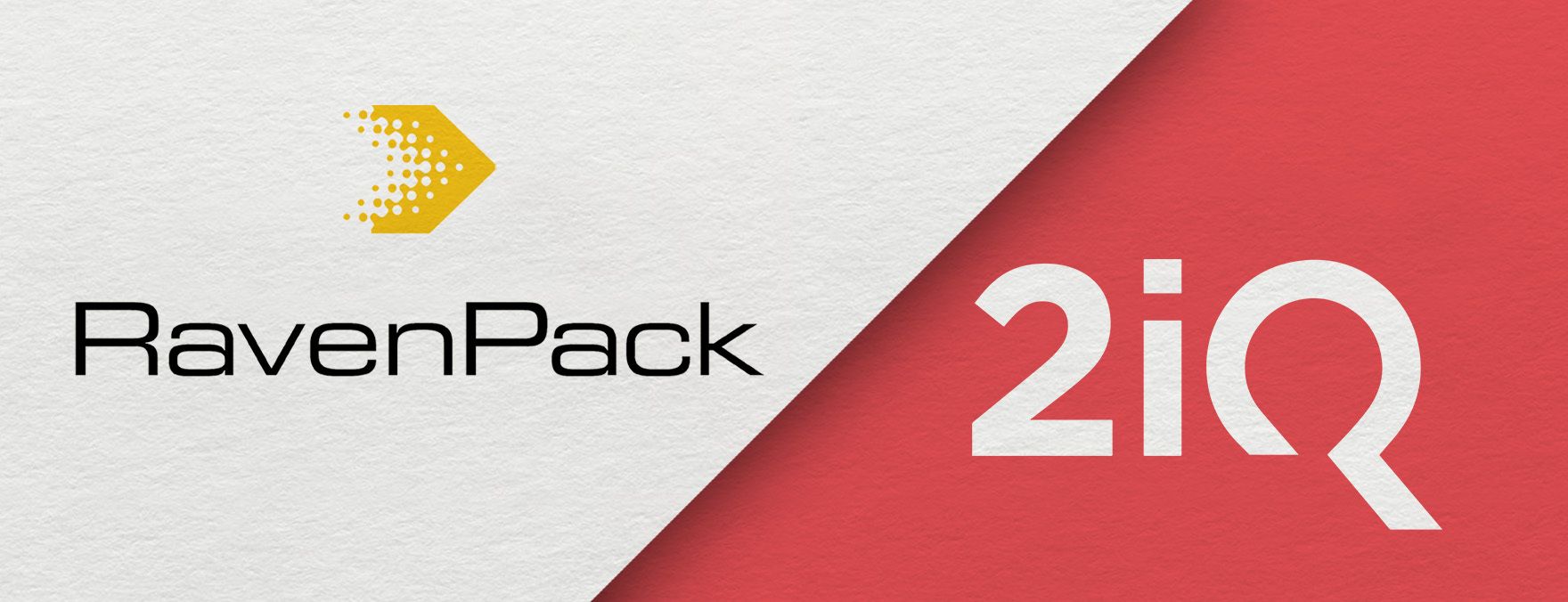
RavenPack is a leading provider of data analytics solutions. Leveraging nearly 20 years of research, along with the power of advanced technologies such as Natural Language Processing (NLP), it helps financial organizations generate actionable insights from unstructured data such as news, social media, earnings call transcripts, and company filings.
Recently, RavenPack used 2iQ Research’s data in a research study focused on creating alpha-generating strategies around the earnings cycle across European markets. The study involved blending three earnings intelligence signals – sentiment from earnings call transcripts, sentiment from earnings-related news events, and net insider trading activity in between reporting periods – to create long-short, dollar-neutral strategies in the European mid/large-cap universe.
Here’s a look at how 2iQ’s insider transaction data was used by RavenPack, and the results from the study.
Creating Alpha-Generating strategies with insider transaction data
The first two data sets RavenPack used in this study were earnings call transcript analytics within RavenPack Edge, sourced from FactSet Transcripts, and earnings-related news analytics, obtained from RavenPack Analytics (RPA 1.0). Here, the company created Document Sentiment signals from the transcripts by aggregating machine learning-generated sentiment of every sentence transcribed from earnings calls. It then combined earnings-related news sentiment with the earnings call transcript signals to develop a more complete picture of earnings cycles.
As for the insider transaction data, the company used RavenPack Insider Transactions, which sources data from 2iQ. This consists of insider transaction data for over 50,000 stocks across 59 countries with an average of 13 years of history across regions. In total, it includes 8.1+ million transactions by over 450,000 insiders, traceable with a unique insider ID, and other relevant features, such as event tagging (M&A, private placements, etc.) and harmonization for insider roles.
To identify the most relevant insider transactions, RavenPack filtered for ‘Insider Level’ ranging from 1 to 4, and on the highest ‘Transaction Significance’ of level 3. The Insider Level analytic describes the relative importance of an insider. For example, level 1 insiders include only top-level positions, such as the CEO, CFO, COO, and Chairman; level 2 identifies group-level executives, such as general counsels, managing directors, and vice presidents; level 3 extends to director-level titles, as well as large hedge fund and private equity holders; and level 4 includes other lower-level executives.
Since corporate insiders cannot trade on actionable non-public information within a legally predetermined period, RavenPack constructed quarterly insider signals by aggregating all relevant insider trades executed between consecutive earnings announcements. This allowed the firm to capture the information contained in those trades during the corresponding reporting period, up to the point it received the transcripts data.
Using the three data sets, RavenPack constructed long-short, dollar-neutral portfolios in the European mid/large-cap universe, normalizing the long and short legs of the portfolio separately to reach 50% long and 50% short exposures. It applied a maximum allocation constraint of 5% per stock using an iterative reallocation process whereby entities that did not reach this constraint were allocated additional capital, proportional to the signal, until maximum gross exposure of 100% was achieved.
Its backtesting period spanned from January 2007 through January 2022. For simplicity, it did not consider trading costs in its analysis. It sequentially introduced the relevant components, starting with the earnings call transcripts, then the earnings-related news, and concluding with insider transactions.
Insider transaction data can help capture Alpha
In terms of the results of the study, RavenPack’s backtests of long-short, dollar-neutral strategies in the European Mid/Large-Cap universe showed that:
Transcript signals based on changes in document sentiment over consecutive reporting periods deliver consistent performance across a wide range of effective holding periods, with an Information Ratio of 1.0 in the Mid/Large-Cap universe, with Annualized Returns ranging from 7% to 3%.
Combining sentiment from earnings-related news with earnings-call transcripts sentiment enhances Information Ratios and Annualized Returns up to monthly effective holding periods. For an effective holding period of ten days, a blended signal improves the Information Ratio of the earnings-news based strategy from 1.3 to 1.5.
Adding an insider transaction overlay to the combined earnings-news and transcript-based signals leads to an approximate 20% increase in Information Ratios for European Large/Mid-Caps over holding periods of more than five days. For an effective holding period of around two weeks, for example, the insider overlay leads to an increase in the Information Ratio from 1.5 to 1.7, with a proportionate improvement in the Annualized Return from 5.8% to 6.4%.
By adding the insider transaction data, RavenPack found significant performance improvements and a slower decay profile relative to the standalone transcript strategies. This was in line with its hypothesis that it should observe different market reactions when executives’ actions matched their words.
In summary, adding the insider transaction data to the earnings news and earnings call transcript data enabled RavenPack to identify stronger, more predictable market reactions.
Commenting on RavenPack’s research study, 2iQ’s Senior Quant, Haris Chalvatzis, said:
“This is a great example of how a research team can utilize insider transaction data to enhance their returns. The authors derive two signals using sentiment from earnings call transcripts and news. In order to further improve them, they utilize transaction data of top-level executives. The beauty of the insider factor is its simplicity. It is purely the difference between the buying and selling activity of the insider, or net insider trading activity. The researchers show that even when they use such a simple measure, they are able to increase the Information Ratio consistently for various holdings periods. To sum up, using the critical information present in the insider data we were able to boost the performance of the underlying signal."
Those interested in finding out more about how RavenPack used 2iQ’s insider transaction data to create alpha-generating strategies across European markets can find additional information here.